Does the repetition of the same category examples result in better learning performance compared to presentation of distinct examples? [publication link]
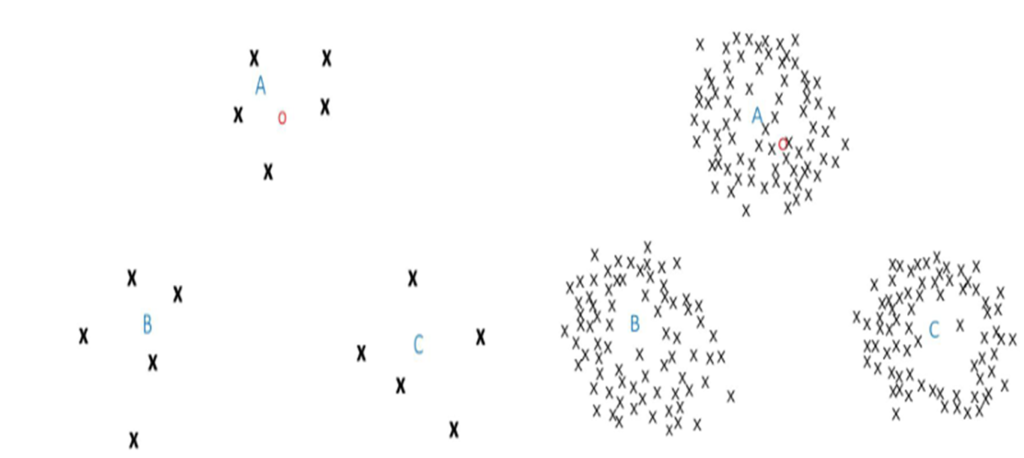
We find that when the training examples are distinct from one other, people are better at generalizing the category knowledge to classify novel items from the same category, even though their ability to tell apart trained and untrained items is not much different for the two training schemes. Through cognitive modeling, we also find that the advantage of distinct examples on category learning can be naturally explained by a mechanism where people make categorization decisions by comparing how similar a new item is to individual examples in memory for each category.
Can learners adjust how much attention they pay to different perceptual features in order to optimize category learning in different contexts? [publication link]
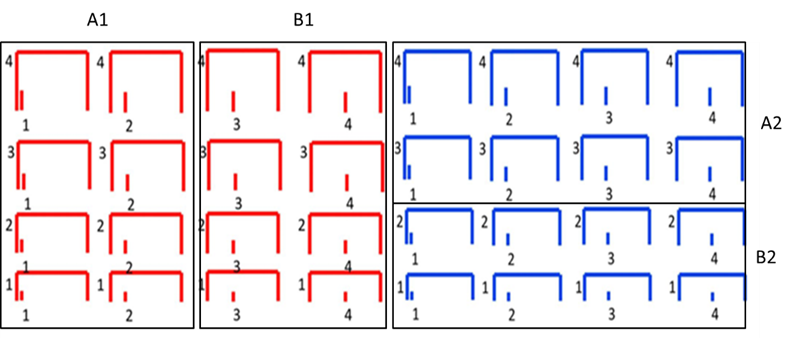
We find that some people are able to learn flexible rules of categorization by focusing on relevant features and ignores irrelevant ones, even when the relevancy of the features change in terms of classifying different training examples. However, we also learned that the flexibility in attention switching across features is only exhibited by learners who tried hard enough to learn the training examples and when some hint of feature relevancy is given (e.g. by labeling sub-categories each with a similar set of features).
Does the diversity of training examples always benefit category learning? [publication link]
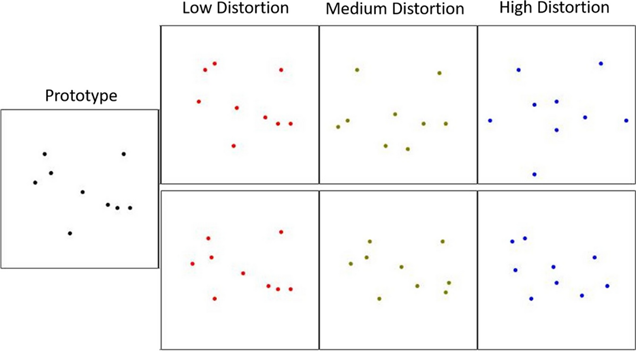
We find that contrary to popular belief, having a diverse set of training examples does not help people generalize category knowledge much better than the same number of less diverse examples. However, we also acknowledge that the counterintuitive finding may be caused by the fact that we use artificially constructed training stimuli (i.e. dot patterns) that have a well-defined, unique prototypical representation, and we cannot make the same claim about natural categories with more complex category structures (e.g. furniture, games).
How can I develop an adaptive example-selection algorithm that selects the training examples on the fly based on individual learning trajectory? Would it be more helpful for category learning if the system just selects the training examples for learners or if the system just gives recommendation to learners when they have the freedom to choose the training examples? [work in progress]
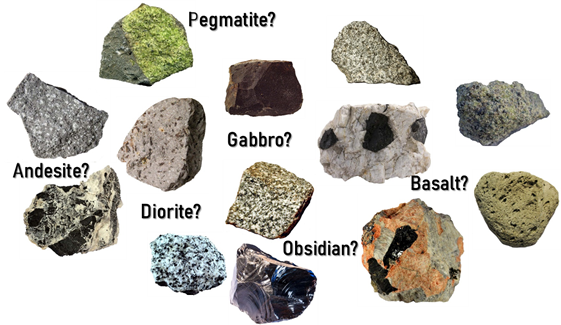
We developed an intelligent tutoring system that automatically adjusts the sequence of training examples based on the classification patterns of individual learners. We find that when training examples are just automatically selected for learners, the ability to classify new examples from the same categories improve at a faster rate and reach a higher level when examples are selected by the system as compared to when examples are evenly sampled from each category. Interestingly, we also find that when given the freedom to choose the training examples, learners tend not to select the examples in a way similar to that of the system, which leads to worse classification performance than if they passively receive examples determined by the system. Even when the system-selected examples are incorporated as recommendations, there are still a fair number of learners who decide not to follow the recommendation and ends up with worse performance.